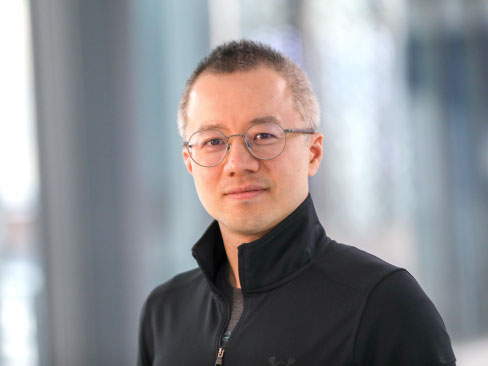
Le Song
Chief Technology Officer
GenBio AI
Professor
Mohamed bin Zayed University of AI (MBZUAI)
Research
Le Song is the CTO of GenBio AI, and also a full professor of Mohamed bin Zayed University of AI (MBZUAI). He was a tenured associate professor of Georgia Institute of Technology, and the conference program chair of ICML 2022. He is an expert in AI and AI for Science and has won many best paper awards in premium AI conferences such as NeurIPS, ICML and AISTATS. Recently, his work on using large language models for protein structure predictions has been featured as the cover story in Nature Machine Intelligence.
Publications
Tutorials and Workshops
- Simon Institute 2017 Workshop on Computational Challenges in Machine Learning
- Machine Learning Summer School 2016 tutorial Dynamic Processes over Networks: Representation, Modeling, Learning, and Inference.
- KDD 2015 tutorial Diffusion in Social and Information Networks: Research Problems, Probabilistic Models & Machine Learning Methods (with Manuel Gomez Rodriguez)
- ICML 2015 workshop Large-Scale Kernel Learning: challenges and new opportunities
- WWW 2015 tutorial Diffusion in Social and Information Networks: Research Problems, Probabilistic Models & Machine Learning Methods (with Manuel Gomez Rodriguez)
- WWW 2015 workshop Diffusion, Activity and Events in Networks: Models, Methods & Applications (with Manuel Gomez Rodriguez and Hongyuan Zha)
- NIPS 2014 workshop Modern Nonparametrics 3: Automating the Learning Pipeline (with Arthur Gretton, Mladen Kolar, Samory Kpotufe and Han Liu and Zoltan Szabo and Andrew Wilson and Eric Xing)
- NIPS 2012 workshop on the Confluence between Kernel Methods and Graphical Models (with Arthur Gretton and Alex Smola)
- NIPS 2012 workshop on Spectral Algorithms for Latent Variable Models (with Ankur Parikh and Eric Xing)
- ICML 2012 tutorial on Spectral Approaches to Learning Latent Variable Models (with Geoffery Gordon and Byron Boots)
Selected Recent Publications
- X. Chen, S. Li, H. Li, S. Jiang, Y. Qi, and L. Song. Generative adversarial user model for reinforcement learning based recommendation system. In International Conference on Machine Learning (ICML), 2019. PDF
- X. Chen, H. Dai, and L. Song. Particle flow Bayes' rule. In International Conference on Machine Learning (ICML), 2019. PDF
- J. Chen, L. Song, M. Wainwright and M. Jordan. Learning to Explain: An Information-Theoretic Perspective on Model Interpretation. International Conference on Machine Learning (ICML) 2018 PDF
- H. Dai, H. Li, T. Tian, X. Huang, L. Wang, J. Zhu and L. Song. Adversarial Attack on Graph Structured Data. International Conference on Machine Learning (ICML) 2018 PDF
- H. Dai, Y. Tian, B. Dai, S. Skiena and L. Song. Syntax-Directed Variational Autoencoder for Structured data Generation. NIPS 2017 Workshop on Machine Learning for Molecules and Materials, 2017 (BEST PAPER), and ICLR 2018, arxiv, PDF
- H. Dai, E. Khalid, Y. Zhang, B. Dilkina, L. Song. Learning Combinatorial Optimization Algorithms over Graphs, arxiv, PDF
- K. Kawaguchi, B. Xie, L. Song. Deep Semi-random features for nonlinear function approximation, arxiv, PDF
- Y. Wang, E. Theodorou, A. Verma and L. Song. A Stochastic Differential Equation Framework for Guiding Information Diffusion, arxiv, PDF
- H. Dai, Y. Wang, R. Trivedi and L. Song. Recurrent Coevolutionary Feature Embedding Processes for Recommendation, Recsys Workshop on Deep Learning for Recommendation Systems, 2016. PDF (BEST PAPER). (Media coverage link)
- H. Dai, B. Dai, Y. Zhang, S. Li and L. Song. Recurrent Hidden Semi-Markov Model. ICLR 2017. PDF.
- H. Dai, B. Dai and L. Song. Discriminative Embeddings of Latent Variable Models for Structured Data, International Conference on Machine Learning (ICML), 2016. PDF. Related to deep learning for graph and network data, and materials science. (Media coverage link)
- B. Dai, N. He, H. Dai and L. Song. Provable Bayesian Inference via Particle Mirror Descent, Artificial Intelligence and Statistics (AISTATS), 2016. PDF (BEST Student PAPER)
- N. Balcan, Y. Liang, L. Song, D. Woodruff and B. Xie. Distributed Kernel Principal Component Analysis, Knowledge Discovery and Data Mining (KDD), 2016. PDF
- M. Farajtabar, Y. Wang, M. Rodriguez, S. Li, H. Zha and L. Song (2015). COEVOLVE: A Joint Point Process Model for Information Diffusion and Network Co-evolution. Advances in Neural Information Processing Systems 25 (NIPS 2015). PDF
- You, Y., Demmel, J., Czechowski, K., Song, L., and Vuduc, R. CA-SVM: Communication-Avoiding Support Vector Machines on Clusters, IEEE International Parallel & Distributed Processing Symposium (IPDPS), 2015. (BEST PAPER)
- Dai, B., Xie, B., He, N., Liang, Y., Raj, A., Balcan, M., and Song, L. Scalable Kernel Methods via Doubly Stochastic Gradients. Neural Information Processing Systems (NIPS 2014). PDF
- Farajtabar, M., Du, N., Rodriguez, M., Valera, I., Zha, H., and Song, L. Shaping Social Activity by Incentivizing Users. Neural Information Processing Systems (NIPS 2014). PDF
- L. Song, A. Anandkumar, B. Dai and B. Xie. Nonparametric estimation of multi-view latent variable models. International Conference on Machine Learning (ICML 2014). PDF
- N. Daneshmand, M. Rodriguez, L. Song and B. Scholkopf (2014). Estimating diffusion network structure: recovery conditions, sample complexity, and a soft-thresholding algorithm. International Conference on Machine Learning (ICML 2014). PDF
- Du, N., Song, L., Rodriguez, M., and Zha, H., Scalable Influence Estimation for Continuous-Time Diffusion Networks, Neural Information Processing Systems (NIPS), 2013. PDF (BEST PAPER)
- Song, L., Fukumizu, K., and Gretton, A., Kernel Embedding of Conditional Distributions, IEEE Signal Processing Magazine, 2013. PDF
- Fukumizu, K., Song, L., and Gretton, A., Kernel Bayes' Rule, Journal of Machine Learning Researches, 2013. PDF
- E. Khalil, B. Dilkina and L. Song. CuttingEdge: Influence minimization in networks. NIPS Workshop on Frontiers of Network Analysis: Methods, Models, and Applications, 2013. PDF (BEST PAPER)
- Song, L., Gretton, A., Bickson, D., Low, Y., and Guestrin, C., Kernel Belief Propagation, International Conference on Artifical Intelligence and Statistics (AISTATS), 2011. PDF
- Song, L., Boots, B., Siddiqi, S., Gordon, G., and Smola, A., Hilbert Space Embeddings of Hidden Markov Models, International Conference on Machine Learning (ICML), 2010. PDF (BEST PAPER)
- Kolar, M., Song, L., Ahmed, A., and Xing, E., Estimating Time-Varying Networks, Annals of Applied Statistics, 2010. PDF
- Song, L., Kolar, M., and Xing, E., KELLER: Estimating Time-Evolving Interactions between Genes, Bioinformatics (ISMB), pp.i128--i136, 2009. PDF
- Song, L., Smola, A., Gretton, A., Borgwardt, K., and Bedo, J., Supervised Feature Selection via Dependence Estimation, ICML 2007. PDF. JMLR 2012 version. PDF
- Smola, A., Gretton, A., Song, L., and Scholkopf, B. A Hilbert Space Embedding for Distributions, Algorithmic Learning Theory (ALT 2007). PDF
Representative Talks
- Embedding as a Tool for Algorithm Design
- COEVOLVE- A Joint Point Process Model for Info Diffusion and Network Co-evolution
- Dynamic Processes over Networks: Representation, Modeling, Learning, Inference, Optimization and Control
- Dirichlet-Hawkes Processes with Applications to Clustering Continuous-Time Document Streams
- Scalable Kernel Methods via Doubly Stochastic Gradients